Headai’s Technology
From Data to Decision Intelligence
Headai’s advanced AI technology combines semantic computing, cognitive reasoning, and machine learning to transform complex, unstructured data into structured, actionable insights. Built on decades of research, it outperforms traditional models in speed, scalability, and energy efficiency.
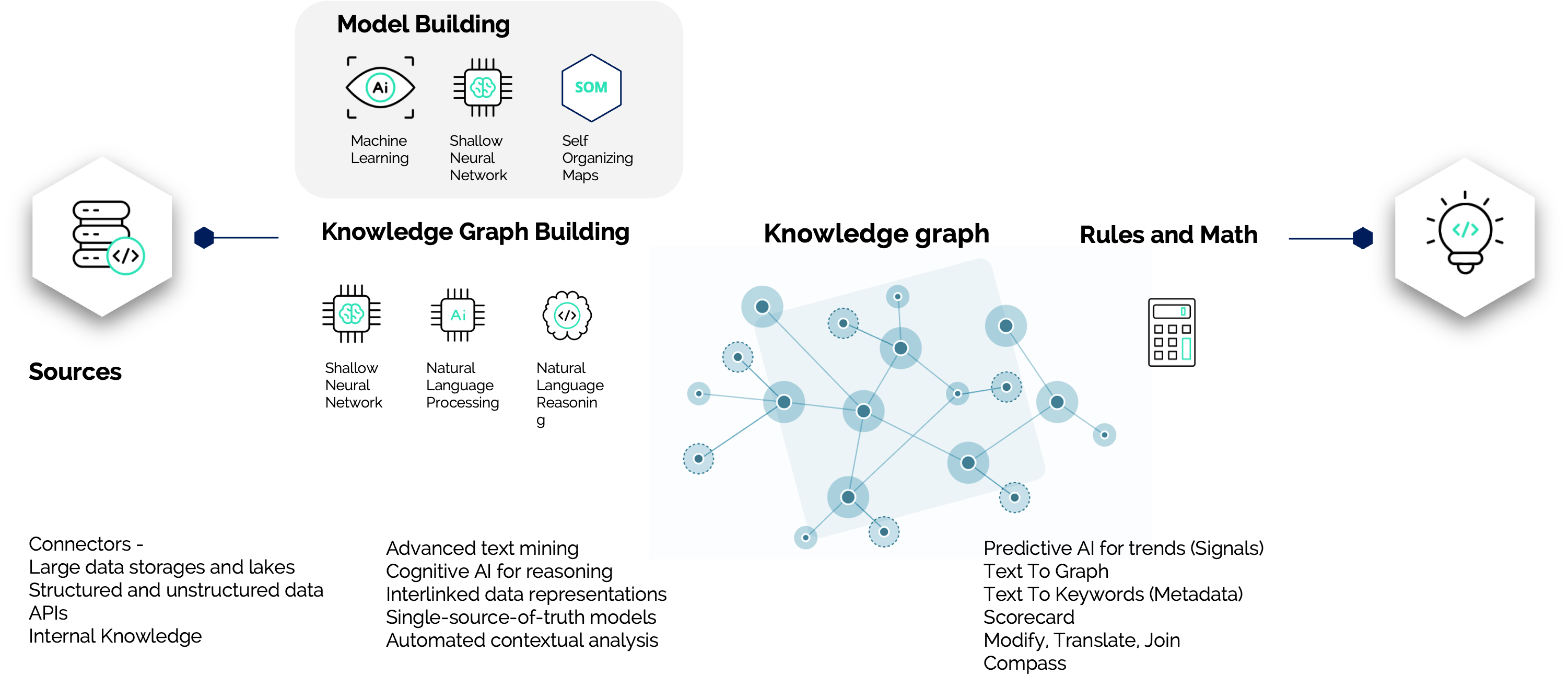
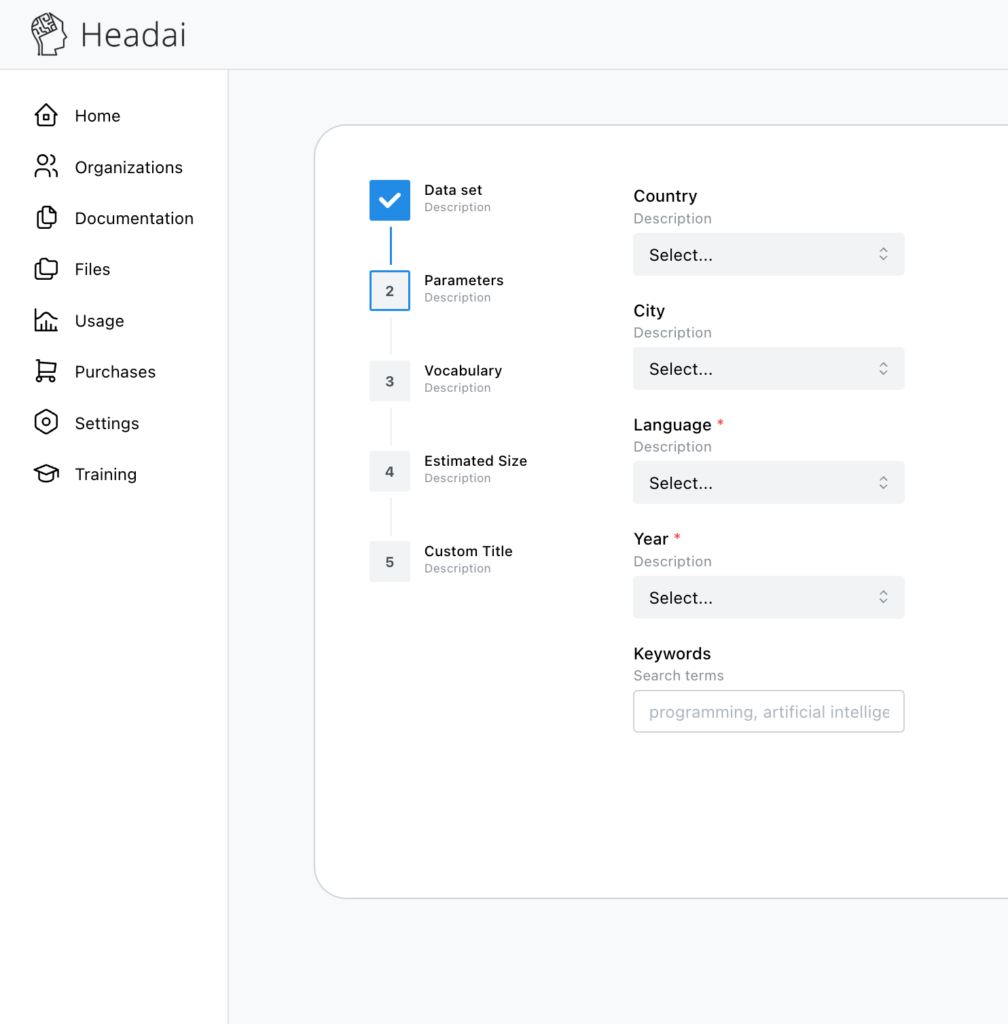
Sources
Where It All Begins
Connectors: Large data lakes like Snowflake, Azure, and AWS.
Structured & Unstructured Data: Databases, reports, APIs, spreadsheets, or freeform text.
Internal Knowledge: Proprietary datasets specific to your organization.
Real-World Data: Headai processes terabytes of information, including scientific articles, job ads, curricula, patents, research reports, and more.
Model Building
Processing and Learning
1.
Self-Organizing Maps (SOM)
Our Self-Organizing Maps offer a lightweight, unsupervised learning method that clusters and organizes data efficiently. Unlike bulky deep learning models, SOMs are compact and resource-friendly, providing actionable insights tailored to specific domains with minimal energy consumption.
2.
Shallow Neural Networks
Headai employs shallow neural networks to process textual data with speed and precision. These models are designed for focused, task-specific operations, ensuring scalability and adaptability without the high computational cost of larger AI systems.
3.
Machine Learning
Headai’s machine learning models identify patterns and make sense of incomplete or domain-specific information. They provide explainable results, ensuring transparency, and perform reliably even with limited data or dynamic environments.
How It Works:
Phase 1
(unsupervised learning), Headai processes general unstructured content (e.g., documents, databases, graphs).
Phase 2
(supervised learning), users teach the AI by validating its performance, improving contextual accuracy for domain-specific tasks.
Don’t know where to start?
Get a free online consultation from one of our in-house experts
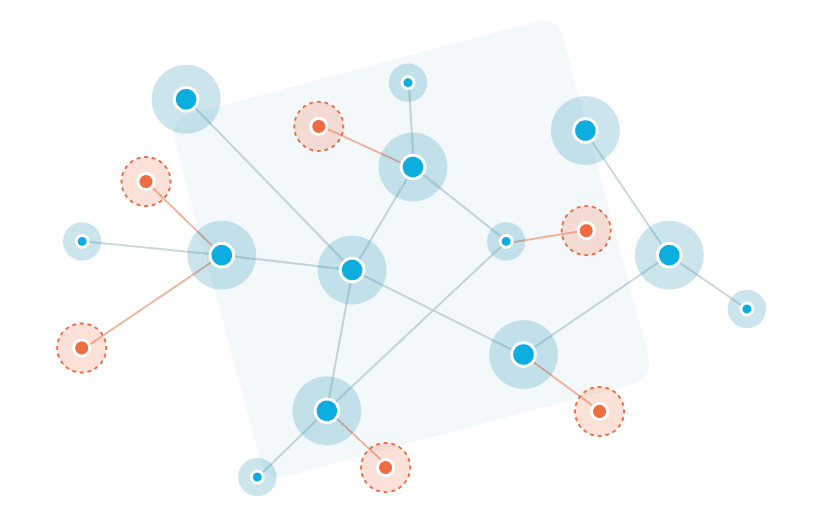
Knowledge Graph Building
Connecting the Dots
Natural Language Processing (NLP): Recognizes words, compound meanings, and keywords from unstructured text.
Natural Language Reasoning: Builds interlinked concepts, mirroring human-like understanding of context.
Advanced Text Mining: Extracts insights, keywords, and relationships to build single-source-of-truth models.
Knowledge Graph Building
Three-Phase Semantic Modeling:
1.
Natural Language Processing (NLP): This process transforms raw textual data into structured formats, to enable subsequent mapping of relationships and insights within the knowledge graph.
2.
Building the Neighbourhood: Words are connected into mind maps to visualize relationships.
3.
Understanding the Relations: Connections are refined and validated to produce actionable insights.
Use cases
World Bank: Analyzed thousands of Kenyan job ads and university curricula to identify skill gaps and labor market demand.
Finnish Defence Forces: Translated military programs into civilian skill language for better career transitions.
Adaptive Learning: Supported global MOOCs and dynamic learning materials with Headai-powered AI.
Functional Capabilities
Headai’s tools address real-world challenges through these core capabilities:
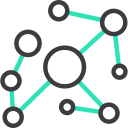
Knowledge Graph
Transform raw data into structured graphs to uncover contextual relationships.
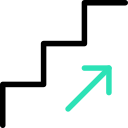
Signals (Predictive AI for Trends)
Detect emerging trends and forecast patterns for strategic planning.
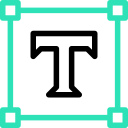
Text to Graph
Generate dynamic graphs from textual data, enabling relationship mapping and exploration.
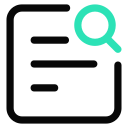
Text to Keywords (Metadata)
Extract and categorize meaningful themes, concepts, and keywords.
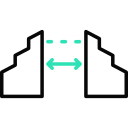
Scorecard
Compare performance against internal KPIs or external benchmarks (e.g., SDGs).
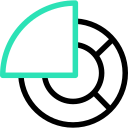
KPI Analysis & Qualitative Balanced Scorecards
Evaluate and optimize qualitative factors of production to monitor strategic goals.
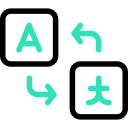
Modify, Translate, Join Knowledge Graphs
Adapt and merge graphs to reflect evolving organizational needs.
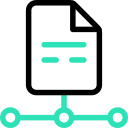
Labeling, Extending, and Organizing Data
Automate organization, extend topics, and add contextual relevance to datasets.
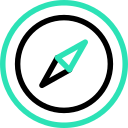
Compass
Simulate outcomes, prioritize actions, and guide skills development or strategic decisions.
Headai Architecture
Headai Core Algorithms: Predictive analytics, simulations, mindmap construction, and scorecards.
Headai Semantic Database: Language semantics, synonyms, compounds, and expressions.
Headai Crawlers: Continuously extract and update real-world data from platforms, systems, and the web.
Customer-Specific Classes: Tailored AI models that address domain-specific challenges.
Why Headai’s Technology Stands Out
Semantic Computing: Models language semantics, enabling deeper, more contextual understanding of data.
Self-Organizing Maps (SOM): Faster, more efficient, and lower energy consumption than traditional deep learning.
Cognitive Reasoning: Handles ambiguous, incomplete, and controversial data to mirror human-like reasoning.
Real-World Applications: Proven success in labor market analyses, curriculum optimization, and adaptive learning systems.